News
Research Highlighted By Chemistry Of Materials Journal
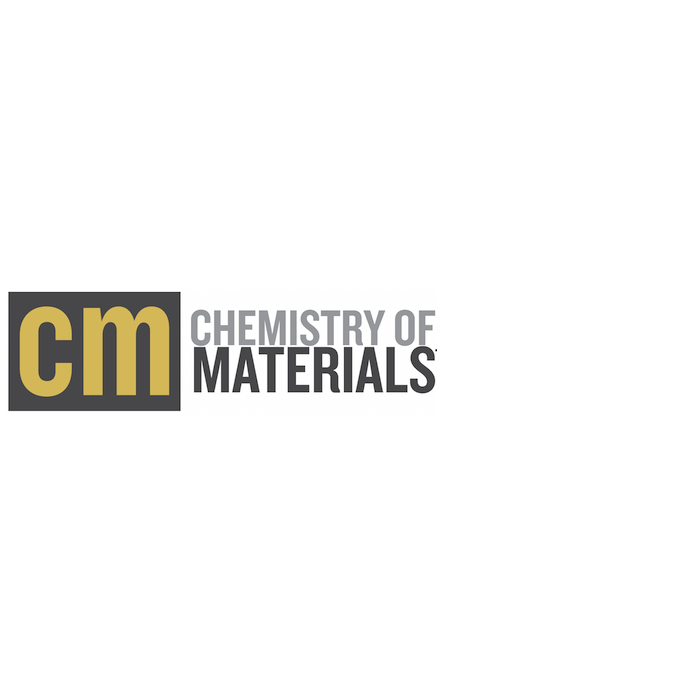
Virtual Issue on Machine-Learning Discoveries in Materials Science
From Chemistry of Materials:
Applications of machine learning, and machine learning-based models in materials chemistry, are a rapidly growing area of research. Traditional methods of exploration are effective but tedious and miss a vast degree of parameter space due to limitations of time and resources. While scientists tend to shy away from using the expression “paradigm shift” due to overuse, data-driven science could actually be the real deal; data-driven science has been termed the fourth paradigm shift after empirical science (the first), model-based theoretical science (the second), and computational science (the third).
Materials science has been a natural area for the growth of data-driven science, as evidenced by the Materials Genome Initiative, and open data resources like The Materials Project. Historically, the great wealth of scientific expertise, experience, and knowledge we take for granted was planned, recorded, and cataloged by previous generations of researchers in scientific reports and articles. The challenge faced today is that, in the era of digitalization of information, it is impossible to process these big data without assistance from computer algorithms. Machine learning enables the discovery of trends in chemical data and provides guidance for new materials via fast screening of unexplored chemical white space. The next ground-breaking discovery of a high-efficiency thermoelectric or a room temperature superconductor may happen thanks to insights from machine learning, and it is exciting to see a growing number of submissions to Chemistry of Materials that view machine learning as a helpful tool for materials discovery. In the current virtual issue, we highlight 22 recent publications, that employ machine-learning methods to target new materials, optimize properties, and predict potentially interesting candidates to synthesize in the laboratory. Accompanying the growth of interest in machine learning is the emergence of newly developed machine-learning methods, which are also reflected in this virtual issue.
Newsletter Registration
Subscribe to our newsletter and stay updated with the latest from Aionics.