Record-Breaking Solid Electrolyte Discovered by Machine Learning, Confirmed by Experiments
News
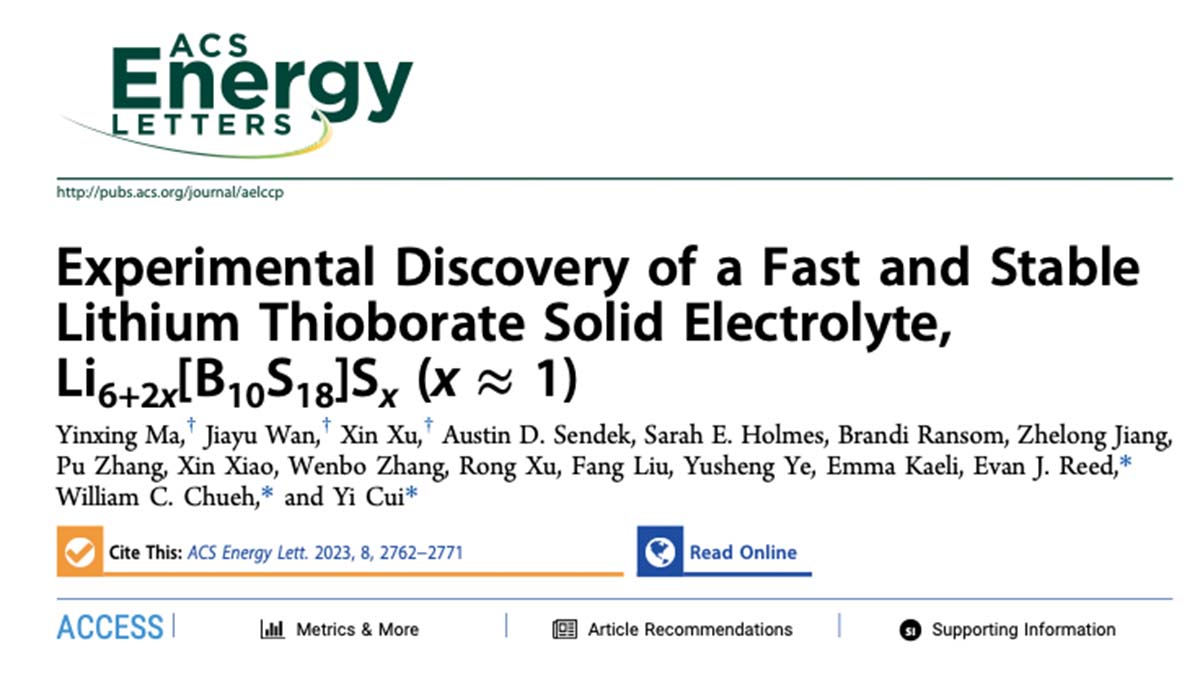
Can machine learning and quantum mechanical simulation techniques actually lead to real discoveries for battery applications? According to a new study in ACS Energy Letters, the answer is yes.
The study, co-authored by Aionics co-founder Austin Sendek and a team from Stanford University, reports experimental measurements on a new solid lithium-ion electrolyte material that appears to break records for performance.
The material is Li8B10S19 — or, more specifically, Li6+2x[B10S18]Sx (x ≈ 1). This lithium thioborate material is a fast lithium ion conductor, with observed room temperature Li-ion conductivity of 0.13 mS/cm — fast enough to serve as a solid electrolyte for solid-state Li-ion batteries.
But where Li8B10S19 really shines is its stability: while most fast ion-conducting materials break down quickly, Li8B10S19 shows extraordinary durability. The team observed an electrochemical window of 1.2 V, a factor of two wider than other fast-conducting sulfide materials. Furthermore, a critical current density of 1 mA/cm2 was observed with a charge/discharge capacity of 1 mAh/cm2. Altogether, this data makes Li8B10S19 the most stable fast Li-ion conducting sulfide ever observed.
This would be an exciting discovery in itself, but the most exciting part of the story is that this material was originally uncovered by machine learning-based screening efforts.
In 2016, a team at Stanford including Sendek and Profs. Evan Reed and Yi Cui built a machine learning model for predicting superionic conduction and used it to screen all 12,000+ known Li-containing materials for the first time. A few interesting lithium-boron-sulfur phases popped up in their final list of the most promising candidates. Two years later, the team published a paper in which they computed the bulk, single-crystal ionic conductivity of ~50 promising candidate electrolytes with density functional theory calculations, including three of the interesting lithium thioborate phases that emerged in the previous study. Then, excited by the promise of these new materials, they examined four lithium thioborate materials in-depth using DFT, computing their ionic conductivities and electrochemical stability windows, and predicting their interfacial reaction pathways on both anodes and cathodes. At this point, it became clear that the lithium thioborate materials were a very exciting class of novel solid electrolyte materials.
In this paper, Sendek, Reed, Cui and co-authors have capped their journey by reporting the successful synthesis of one promising lithium thioborate phase, and observing very positive experimental measurements of its high ionic conductivity and stability.
According to Sendek: “this journey illustrates how valuable computation can be for identifying the proverbial needle in the haystack when the search space of materials is large. Twelve thousand candidates with ML, fifty candidates with high-throughput DFT, four candidates with focused DFT, and one candidate with experimentation.”
Since its founding, Aionics has built and deployed similar machine learning and density functional theory-based tools for conducting large-scale screening for both solid and liquid electrolyte components, for many leading automotive, battery, and chemical companies. To hear more about how Aionics helped co-innovate on liquid electrolyte development with Sepion Technologies, check out Aionics Fortnightly Episode 13 with Dr. Jessica Golden.
The team of co-authors includes Sendek, Reed, Cui, Yinxing Ma, Jiayu Wan, Xin Xu, Sendek, Sarah Holmes, Brandi Ransom, Zhelong Jiang, Pu Zhang, Xin Xiao, Wenbo Zhang, Rong Xu, Fang Liu, Yusheng Ye, Emma Kaeli, and Prof. William Chueh.
Newsletter Registration
Subscribe to our newsletter and stay updated with the latest from Aionics.