Machine Learning with Big and Small Data for Materials Design with Prof. Qian Yang
Past Fortnightly -
While many powerful tools from machine learning have found their way to impactful applications in materials science, it would be a mistake to think of this as a one-way exchange. Increasingly, the unique opportunities and challenges arising from scientific datasets will also have an impact on algorithmic developments in the field of machine learning.
Prof. Qian Yang, Assistant Professor at the University of Connecticut and Aionics Scientific Advisory Board member, is bridging this gap. A computer scientist by training whose doctoral thesis work focused on ML applications in materials science and chemistry, Prof. Yang is committed to expanding the two-way exchange of ideas between the physical sciences and machine learning.
In Aionics Fortnightly Episode 22, Prof. Yang will discuss some examples of how this synergy between fields has evolved in her lab’s work at UConn applying machine learning to materials science and chemistry applications. She will highlight several diverse examples, including data-driven learning and model reduction of nonlinear dynamical systems such as chemical reaction networks, and active learning with model selection.
Her work highlights the importance of carefully designing performance measurement for machine learning models in order to correctly understand their predictive ability — a prerequisite step to leveraging such models to drive real-world impact in the lab.
Newsletter Subscription
Subscribe to our newsletter and stay updated with the latest in material informatics.
Guest bio
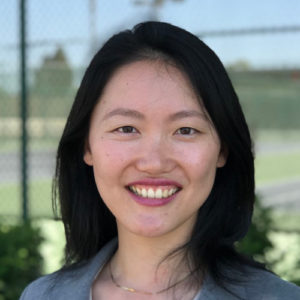
Prof. Qian Yang
Assistant Professor, Computer Science & Engineering Department, University Of Connecticut
Qian Yang is an Assistant Professor in the Computer Science and Engineering Department, and affiliated with the Institute of Advanced Systems Engineering, at the University of Connecticut. She holds a Ph.D. from Stanford University, and a B.A. from Harvard College. Her research interests lie at the intersection of computational science and the physical sciences, with an emphasis on machine learning for materials, physics, and chemistry applications. She is also the organizer and co-host of Materials and Megabytes, a podcast on machine learning for materials science.