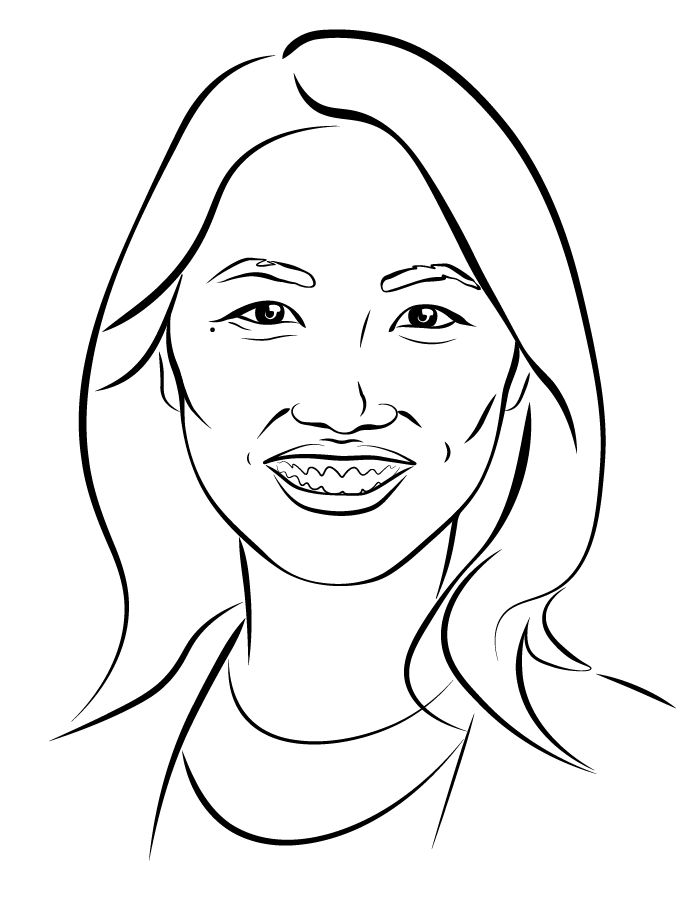
Past Fortnightly
Interpretable Models for the Physical World with Prof. Qian Yang
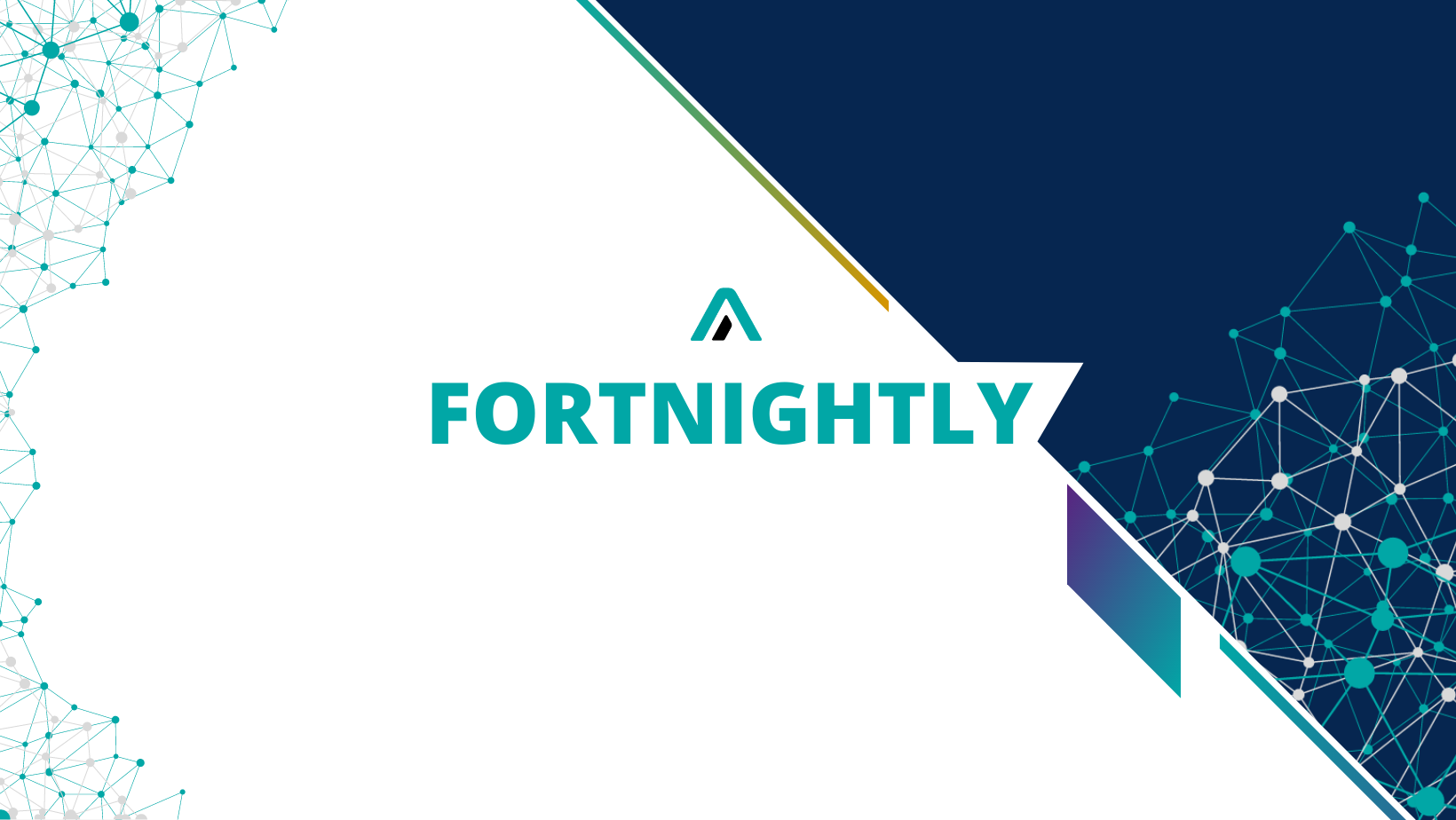
Two important challenges in scientific machine learning are interpretability – the ability to understand how and why models are making their predictions, and extrapolability – the ability to make predictions outside the data regime that the model was trained on. In the field of scientific machine learning, one can often leverage problem structure to address these challenges. In essence, having a scientific understanding of a problem enables us to “prime” the machine learning algorithm. This is important in materials science and chemistry, where practitioners may have a limited, but nonzero, understanding of how a system behaves.
In this talk, Prof. Qian Yang of the University of Connecticut will discuss three examples of how hierarchical structure and invariances can be used to build predictive machine learning models for materials properties. The first two examples use graph neural networks to automatically learn how substructures affect materials properties, and to learn properties of mixtures. Finally, the third example uses a hierarchical method for multi-class classification to recover structural information from experimental small angle scattering curves.
Join us for our reboot of Aionics Fortnightly on Thursday, August 17 at 10am PDT!
Newsletter Subscription
Subscribe to our newsletter and stay updated with the latest in material informatics.
Guest bio
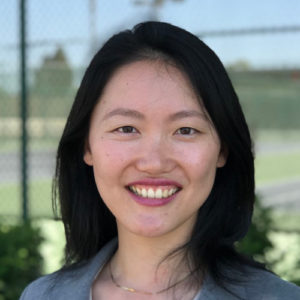
Qian Yang
Assistant Professor, Computer Science & Engineering at the University of Connecticut
Qian Yang is an Assistant Professor in the Computer Science and Engineering Department at the University of Connecticut. She received her Ph.D. in Computational and Mathematical Engineering from Stanford University, and holds a B.A. in applied mathematics with computer science focus from Harvard College. She has an interdisciplinary background in machine learning and computational materials science and at UConn her lab’s research interests lie at the intersection of these fields, with the ultimate goal of enabling scientific discovery through new data-driven paradigms for modeling and computation.