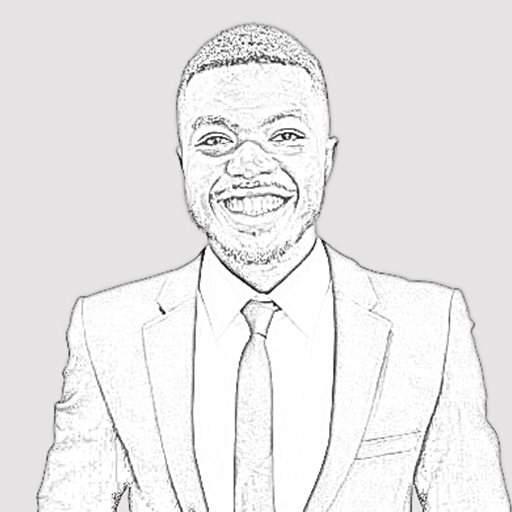
Past Fortnightly
Data-driven electrolyte design for lithium metal batteries with Dr. Solomon Oyakhire
Improving Coulombic efficiency (CE) is key to the adoption of high energy density lithium metal batteries. Liquid electrolyte engineering has emerged as a promising strategy for improving CE, but its inherent complexity makes CE prediction and electrolyte design challenging. In this talk, I will introduce machine learning (ML) models and workflows that inform the design of high-performance electrolytes.
Using the elemental composition of electrolytes as the features of our models, we apply linear regression, random forest, and bagging models to identify the critical features for predicting CE.
Our models reveal that a reduction in the solvent oxygen content is important for attaining high CE. Equipped with this insight and a few others, we formulated five new electrolyte compositions with fluorine-free solvents, one of which attains a high CE of 99.70%.
Altogether, this work underscores the promise of data-driven approaches in expediting the discovery of high-performance electrolytes for lithium metal batteries.
Newsletter Subscription
Subscribe to our newsletter and stay updated with the latest in material informatics.
Guest bio
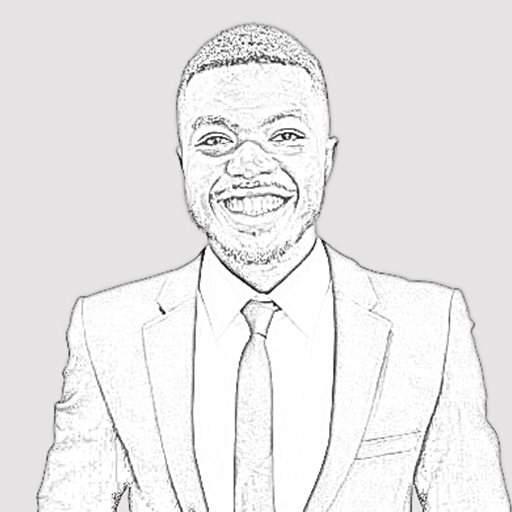
Dr. Solomon Oyakhire
Schmidt Science Postdoctoral Fellow at UC Berkeley
Solomon is a Schmidt Science postdoctoral fellow at UC Berkeley where he is advised by Professor Kristin Persson and Professor Gerbrand Ceder. He received his PhD in Chemical Engineering at Stanford University, where he was advised by Professor Stacey Bent and Professor Yi Cui. His research is focused on using surface science and machine learning for understanding reactivity and electron transfer in energy systems. Prior to Stanford, he received his BSc. in Chemical Engineering with highest honors from the University of Lagos, Nigeria.