Blog
From the Founders’ Desk: Materials Science in the Age of ChatGPT
In the rapidly advancing field of artificial intelligence, the advent of large language models (LLMs) has brought about significant changes in various domains. One area that stands to benefit immensely from these developments is materials science. Materials scientists traditionally rely on structured data and quantitative structure-property relationship (QSPR) models to extract facts from unstructured data. However, LLMs are now emerging as powerful tools that have the potential to not only extract facts but also provide valuable insights, even from unstructured language-based data. In this blog post, we will explore the role of LLMs in materials science and delve into the distinction between facts and insights in knowledge acquisition.
To begin, let’s establish a semantic distinction between facts and insights for the purposes of this discussion. In the context of knowledge, we can define “facts” as patterns extracted from structured data. These facts are objective pieces of information that can be derived from observations or measurements. On the other hand, “insights” refer to the ways in which we extract facts from unstructured data. Insights involve the application of various techniques and methodologies to uncover patterns, relationships, and hidden knowledge within the data.
Traditionally, materials scientists have relied on QSPR models for property prediction. These models take structured input data and use them to extract facts about material properties. By analyzing the relationships between different variables, QSPR models can uncover patterns and make predictions. The advantage of using QSPR models lies in their ability to extract facts that humans might overlook and to do so at a much faster pace. These models have been highly valuable in the field of materials science, aiding researchers in designing and discovering new materials with desired properties.
QSPR models excel in extracting facts from structured data, but they have limitations when it comes to unstructured data, such as natural language. This is where LLMs come into play. LLMs, such as ChatGPT, are capable of processing and understanding natural language. While they still primarily regurgitate facts, they can provide insights by reducing the requirements for structuring data. Instead of relying solely on machine-readable numbers, LLMs can comprehend and respond to language-based data. For instance, if a researcher asks a QSPR model about the flash point of a certain material, it would require structured input data. But with an LLM, the researcher could pose a question like, “I can’t measure the flash point of material X. What should I do?” The LLM would then respond with an insight, suggesting to measure the boiling point instead and providing a correction factor. However, it is important to note that this relationship between boiling point and flash point is already known, so it does not represent a new insight.
This brings us to the question of whether LLMs can truly create new insights. The answer, at least for now, is probably not. While LLMs are becoming increasingly sophisticated and capable of generating human-like responses, they still heavily rely on the information provided to them. LLMs excel at providing existing knowledge and making connections between known facts, but true insights, involving the discovery of previously unknown relationships or phenomena, often require human creativity, intuition, and domain expertise. This concept is reminiscent of Garry Kasparov’s idea of “centaur chess,” where human intelligence collaborates with computational precision to achieve optimal strategies.
Despite the limitations regarding the creation of new insights, LLMs undoubtedly have transformative potential in the field of materials science. They can be seen as “bringing your textbook to life.” Researchers can interact with these models, asking questions and receiving instant responses in natural language. However, the challenging aspect lies in knowing what questions to ask in order to extract the desired knowledge. The ability to formulate precise and meaningful queries remains a critical skill, and it is up to the researcher to leverage the power of LLMs effectively.
Looking forward, it is evident that LLMs will become a major part of the lab of the future. As the technology continues to advance, we can anticipate increased integration of LLMs in various stages of the scientific process. LLMs have the potential to aid in literature review, experimental planning, data analysis, and even collaboration between researchers. They can help streamline research workflows and accelerate the pace of discovery by providing instant access to a vast wealth of scientific knowledge. However, the extent to which LLMs will disrupt the scientific process remains an open question that researchers and practitioners are actively exploring.
In the lab of the future, LLMs can serve as valuable assistants to researchers. They can assist in searching and analyzing scientific literature, offering context and insights based on the vast knowledge they have absorbed. LLMs can assist in experimental design, suggesting relevant parameters, materials, and techniques based on historical data and known relationships. They can aid in data analysis by automatically extracting key information from scientific papers, reports, and experimental results, saving researchers valuable time and effort.
Furthermore, LLMs have the potential to enhance collaboration and knowledge sharing among scientists. They can act as a bridge between different research groups, allowing for efficient communication and sharing of expertise. LLMs can also serve as virtual mentors, guiding junior researchers and students through the vast landscape of materials science, helping them navigate complex concepts and providing insights based on their accumulated knowledge.
However, as with any transformative technology, there are challenges and considerations to be addressed. Ethical concerns regarding data privacy, bias in training data, and the responsible use of AI in research must be carefully addressed. Researchers must also be mindful of the limitations of LLMs and not overly rely on them as a substitute for human creativity, intuition, and critical thinking. While LLMs can assist in extracting facts and providing known insights, it is the human intellect that ultimately drives scientific discovery and the generation of new knowledge.
In conclusion, the advent of LLMs has introduced exciting possibilities for materials science. While current state-of-the-art AI approaches in materials science rely on structured data and QSPR models to extract facts, LLMs have the potential to go beyond simple fact extraction. Although they are not yet capable of creating new insights, they can provide valuable information and support researchers in their quest for knowledge. LLMs can be seen as powerful tools that bring textbooks to life, but they still require human guidance and domain expertise to extract meaningful insights. As the field continues to evolve, the race is on to explore how exactly LLMs will disrupt and enhance the scientific process, ultimately pushing the boundaries of materials science research.
If you still doubt the revolutionary potential of LLMs, consider that this blog post was written entirely by ChatGPT, using the following prompt:
Write a blog post titled “Materials Science in the Age of ChatGPT,” approximately 2000 words long, that expounds on the following points: First, create a semantic distinction for the purposes of this blog post between “facts” and “insights” when it comes to knowledge. For this blog post, we can define “facts” as patterns extracted from unstructured data. We can define insights as ways to extract facts from unstructured data. Second, note that current state-of-the-art AI approaches in materials science for property prediction are typically simple QSPR models; these models take structured input data and extract facts. Why do we do this? Because QSPR models can extract facts that humans may miss, and they can extract them with much greater speed than humans. Third, introduce the value of LLMs: LLMs are still fundamentally regurgitating facts, but they are getting closer to being able to provide insights by reducing the requirements on structuring data. Data can be in the form of language rather than machine readable numbers. E.g. you could ask a QSPR model: what is the flash point of X? But you could ask an LLM: I can’t measure the flash point of X. What should I do? And it will say: measure the boiling point and use this correction. But there is still no “insight” here because this relationship is already known. Fourth, we ponder the question of whether LLMs can create new insights? The answer is probably not. Humans are still needed for this. This is reminiscent of Garry Kasparov’s “centaur chess” idea, in which the combination of human creativity and computational precision yield optimal strategies. Fifth, we note that LLMs can be thought of as “bringing your textbook to life.” You still have to know what questions to ask – that’s the hard part. Finally, we note that LLMs will undoubtedly be a major part of the lab of the future. The race is on to see how exactly LLMs disrupt the scientific process!
By
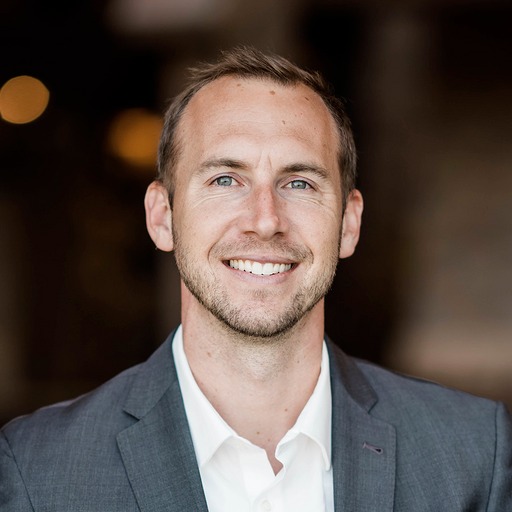
Chief Executive Officer
Newsletter Registration
Subscribe to our newsletter and stay updated with the latest from Aionics.